Masha Naslidnyk
📍 PhD student @ UCL. She/her.
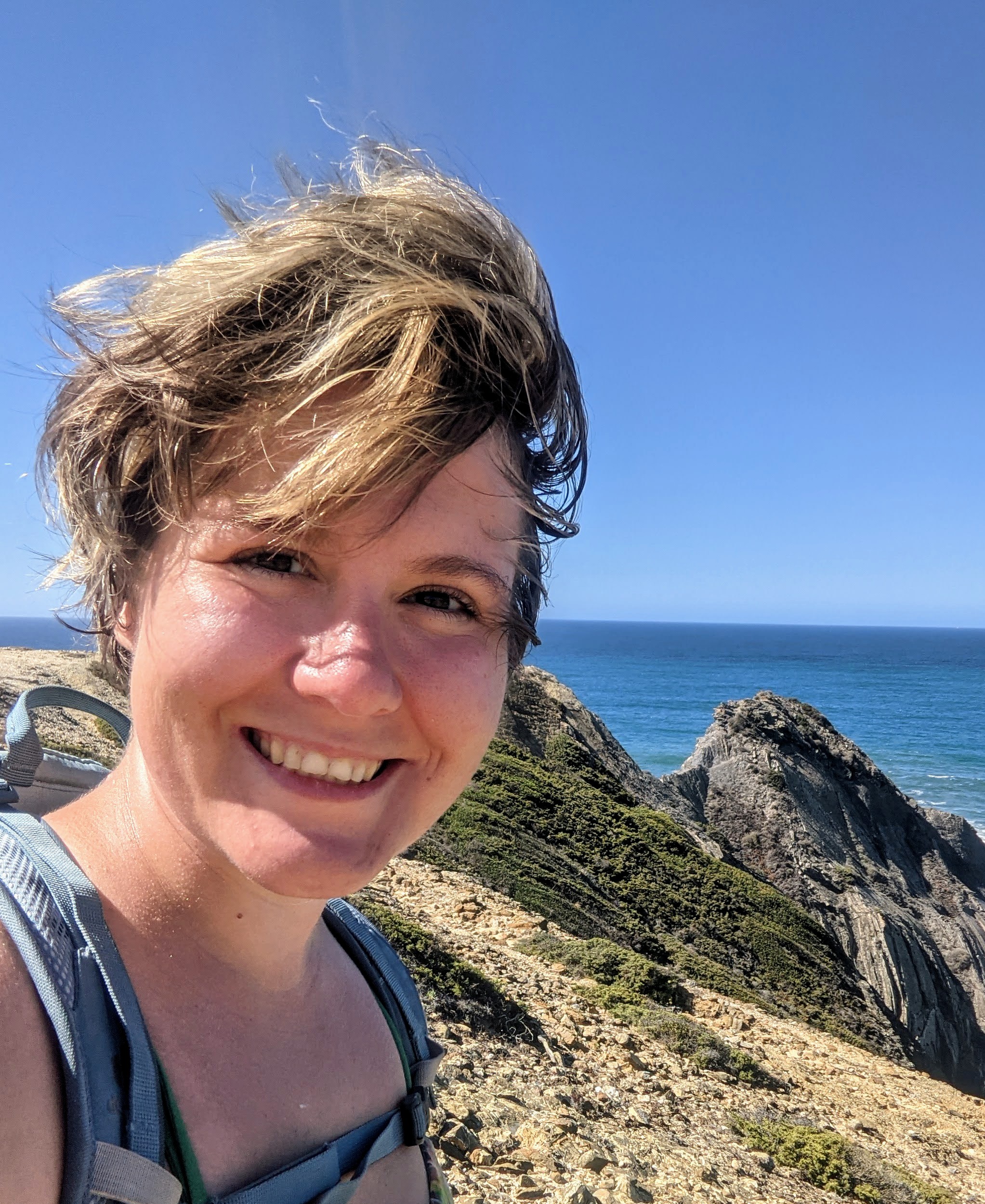
Hi! I am a PhD student at the Fundamentals of Statistical Machine Learning research group and the Foundational AI CDT at University College London, advised by F-X Briol, Jeremias Knoblauch, and Carlo Ciliberto. Prior to starting my PhD, I was a Machine Learning Scientist at Amazon Research in Cambridge, where I worked on Alexa question answering (2015-2019), and then on Gaussian processes for supply chain emulation (2019-2021). I graduated from Part III in Pure Mathematics at the University of Cambridge in 2014.
My research interests lie broadly in the topics in Gaussian processes and kernel methods; at present, I am focussed on robust inference in conditional probability models.
I will happily respond to “Masha”, but if you’d like to pronounce my last name, it’s nah-sleed-nyk.
news
Jul 01, 2024 | đź’¬ Giving a talk at the 2024 ISBA World Meeting in Venice, Italy. |
---|---|
Sep 01, 2023 | 🌍 Visiting CISPA Helmholtz Center for Information Security under the Helmholtz Visiting Researcher Grant, September-November 2023. |
May 08, 2023 | đź’¬ Co-organising the Distance-based methods in Machine Learning workshop. |
Mar 29, 2023 | đź’¬ Our paper Optimally-Weighted Estimators of the Maximum Mean Discrepancy for Likelihood-Free Inference was accepted at ICML 2023. |
Jan 12, 2023 | 🎉 An upcoming paper, Robust Empirical Bayes for Gaussian Processes, won an ASA Section on Bayesian Statistical Science (SBSS) student paper award. It will be presented at JSM 2023. |
papers
* indicates equal contribution.- EMNLP-IJCNLPUsing Pairwise Occurrence Information to Improve Knowledge Graph Completion on Large-Scale DatasetsIn Proceedings of the 2019 Conference on Empirical Methods in Natural Language Processing and the 9th International Joint Conference on Natural Language Processing (EMNLP-IJCNLP), 2019